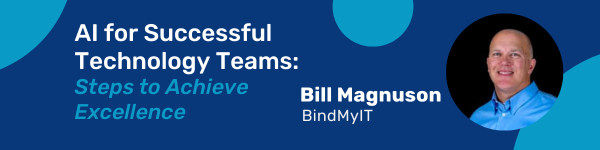
In today's fast-paced technology landscape, the integration of Artificial Intelligence (AI) has become a game-changer for businesses seeking to enhance internal and external customer experiences. For professionals well-versed in various aspects of the technology industry, understanding the simplicity of AI and the crucial steps required for success can be a significant advantage. In this blog post, we'll delve into the core concepts of AI, outline the key steps for technology teams to excel, and offer valuable tips for getting data sources right.
The Simplicity of AI
Despite its reputation for complexity, AI can be simplified into its fundamental components. At its core, AI is about teaching machines to learn from data and make intelligent decisions. For our audience of technology professionals, this means understanding that AI isn't magic, but a tool driven by algorithms and data.
Key Components of AI:
1. Data: Data is the lifeblood of AI. Accurate, relevant, and diverse data is essential for training AI models. Ensure that your data sources are comprehensive and up to date.
2. Algorithms: Algorithms are the brains behind AI. These mathematical instructions process data and make predictions or decisions. Familiarize your team with various AI algorithms and their applications.
3. Model Training: Training AI models involves exposing them to data to learn patterns and make predictions. It's an iterative process that requires constant refinement. It is very important that the models being trained are consistent with your organization’s culture.
4. Evaluation and Testing: Rigorous testing is crucial to ensure AI models perform as expected. Monitor their performance and fine-tune them as needed. Make sure that you include your customers in the evaluation and testing process.
Steps for Technology Teams to Succeed with AI
1. Define Clear Objectives: Start by defining your AI project's objectives. What specific problem are you trying to solve, and how will AI help achieve this? Clear goals are essential.
2. Assemble the Right Team: Form a cross-functional team with data science, software engineering, and domain knowledge expertise. Collaboration is key.
3. Data Quality Matters: Garbage in, garbage out. Ensure your data is clean, relevant, and well-structured. Invest in data quality tools if necessary.
4. Choose the Right Tools: Select AI frameworks, libraries, and tools that align with your project's requirements. Consider open-source options and cloud platforms.
5. Iterate and Improve: AI is an ongoing journey. Continuously evaluate and refine your models to adapt to changing conditions and data.
Tips for Getting Data Sources Right
1. Data Diversity: Gather data from diverse sources to minimize bias and enhance model performance.
2. Data Governance: Establish clear data governance policies to ensure data integrity, security, and compliance.
3. Data Preprocessing: Clean, preprocess, and normalize data before feeding it to AI models to improve accuracy.
4. Feature Engineering: Craft meaningful features from raw data to enable better model insights.
5. Data Validation: Implement rigorous data validation processes to detect and rectify errors early on.
In conclusion, AI need not be shrouded in mystery for technology professionals. By understanding its core components, following the right steps, and paying attention to data quality, your technology team can harness the power of AI to deliver exceptional internal and external customer experiences. Stay informed, collaborate effectively, and embrace the AI revolution to stay ahead in the ever-evolving technology industry.
Remember, AI is not just a buzzword—it's a transformative force, and you have the knowledge and tools to leverage it effectively.
Bill Magnuson is the co-founder and CEO of BindMyIT, a leading technology solution consulting company. With over 26 years of experience in the tech industry, Bill is passionate about harnessing AI's potential to drive innovation and improve customer experiences.